Top 8 Data Science Use Cases in Sales
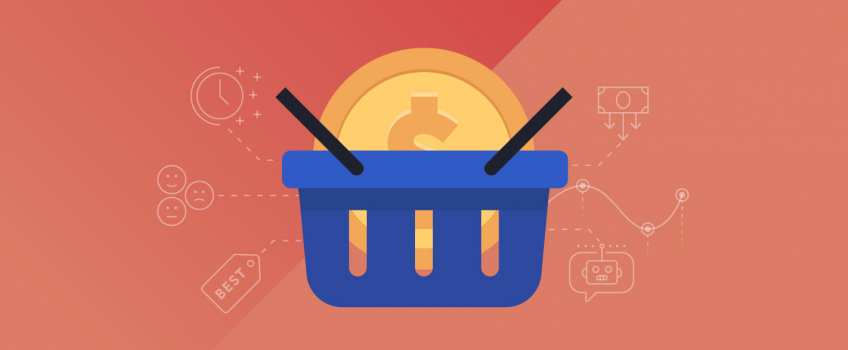
In the times when data is of extreme value, industries cannot afford to ignore it. The only right choice for them is to find new ways to use data for their benefit.
These words have become a motto for the sales industry along with the others. As far as the feature of the repetitiveness may characterize sales industry, there are numerous dimensions where data science may be applied. All in all, data science brings growth, improvements, efficiency, and effectiveness in sales. Thus, the collective dream of all those dealing with sales - to sell more with fewer efforts becomes real with the use of Data Science tricks.
Therefore, the only thing left is to choose the sphere of high potential to get the most of it. That is why we decided to make a list of the most frequently used and thus the most efficient use case of data science in sales.
Customer sentiment analysis
Customer sentiment analysis serves as a tool to extract emotional intelligence from communication. Thus, we can easily understand the emotions and use this understanding for the benefit of our business.
Sentiment analysis relies on the text mining algorithms which allow studying the general attitude towards the texts available via social media platforms, blogs or review sites. Automated sentiment analysis tools allow getting a real-time actionable insight in one click.
These tools highlight the subtext of the comments taking into account information, mood, and general opinion. Besides the general classification into positive, negative or neutral comments, the extent of these emotions may be identified as well.
The customers' feedback is where you should search for the improvements. Application of the customer sentiment analysis tools is unavoidable in case you are to understand what customers want when they want it, and why they want it.
Maximization of customer lifetime value (CLV)
Customer lifetime value is a key metric for smart business decisions. The CLV reflects the profit a customer brings over the entire period of interaction with the brand. Knowing your customers' lifetime value helps you to get a general overview of your business perspectives in future. A number of indicators are used involved here, such as gross margin, purchase frequency, average order value, etc. Smart algorithms totally take care of tracking changes in the data and measuring, comparing and calculating them.
Having all these metrics at hand opens a fabulous opportunity to maximize the lifetime value of your customers. Here come personalized recommendations, personalized newsletters campaigns, customer loyalty programs. These actions are called upon to increase the metrics. The steps are simple:
-
compare your metrics
-
take actions
-
define next weak metrics
and repeat starting with Step 1.
Future sales prediction
Prediction of future sales brings great relief to the companies working with sales. Those who sell - have stock and they need to manage it wisely. In case, there are too many items of one product on stock they risk not to have enough space for other goods or to be forced to sell at a discount. On the contrary, the sales fall when the items are too few. Prediction of future sales allows them to avoid these problems and make better decisions.
The prediction model requires particular data. Here belongs the number of customers gained, the number of customers lost, the average level of sales along with the seasonal tendencies. Also, the sales assumptions - changing circumstances that can significantly affect sales - should be predetermined.
Sales forecasting algorithms look for patterns in this data. The detected patterns are further used to score the general tendencies of the deals in the pipeline to build predictions with a high level of accuracy.
Churn prevention
Now when the sales market players have the tools to predict when a customer is going to make the next purchase, one more important thing to do is to predict when a customer is going to stop buying.
Customer churn is the term used to refer to the percentage of customers who stopped buying the product and ended its use during a particular period. Machine learning algorithms go through the Customer Relationship Management data to spot the patterns and characteristic features in the behavior, communication, and ordering of those, who stopped buying. In comparison to the characteristics and periodical changes in the behavior of the present customers, it is quite easy to determine those who are likely to end up the relationship with a brand. The reasons for customer churn may be various: price, product fit, unsatisfactory customer experience, etc. To prevent or at least to reduce customer churn try to lean on your best customers, provide feedback and prompt communication, offer bonuses, ask about your customers’ opinions and take it into account.
Cross-sell recommendations
Cross-sell recommendations help to retain the customers and make their relationships with a brand longer. Smart data tools provide an opportunity to tailor personalized recommendations which proved to be a robust up-sell tool. Cross-selling presupposes offering of the additional item to a customer who has already bought something or intends to buy.
The algorithm goes through sale transaction data and generates rules demonstrating the percentage of times the products are bought together. Thus, the role of data science is to provide fact-based recommendations from CRM data and transaction data.
These algorithms help to decide which products may be offered together or even placed on the same page in the catalog. Cross-selling also involves package deals. The analysis results help to form packages of the items offered at a discount.
Price optimization
Setting the right price is one of the most challenging tasks of all time. The price should be satisfactory both for those who sell and those who buy. This balance is quite hard to reach. There are numerous pricing strategies which may be used for this task. Data science made its way to set prices and improved this process significantly. Do algorithms help to evaluate the potential sales promotions and estimate the right price?
Price optimization models calculate how demand varies at different price levels in comparison to the costs of production and stock to make a right price. These models are also used to tailor prices for particular customer segments.
Price optimization directly influences on customers' satisfaction rates. Moreover, the pricing policy predetermines the type of customers you attract, how your brand is perceived and of course the amount of your profit.
Chatbots - salespeople
Using bots instead of salespeople seems to be the most exciting data science use case in sales. Chatbots contribute to the automation of interactions with customers and reduce the time spent on problem-solving.
Modern chatbots are empowered by sentiment analysis algorithms for a better understanding of customers' messages. Moreover, chatbots are capable of simultaneous conversations and sending hundreds of messages per minute. Thus, the efficiency of sales bots is extremely high. In some cases, chatbots proved to provide better customer experience, as they process the inquiries instantly.
Key benefit of hiring a sales bot is that it saves money.
Implementation of Augmented Reality (AR)
Augmented Reality has a tremendous perspective on implementation in the sales industry. The use of Augmented Reality can provide customers with the far more realistic shopping experience, especially when it comes to online stores.
First of all, Augmented Reality may be used to improve navigation among the products and shelves both in real shops or online platforms. Secondly, the availability of virtual fitting rooms. Customers get an opportunity to interact with a product, which increases the chances to buy it.
AR encompasses the elements of gamification, entertainment, visualization. Thus, the last but not the least, is that Augmented Reality provides brighter emotions and feelings, and unforgettable shopping experience to customers. New exciting experience always motivates people to buy more.
Conclusion
Without any doubt, data science brings its positive effects to all industries. Any industry can benefit from data-based, well-structured and highly-accurate decisions. Taking into account all the instances provided in our article, the sales industry actively applies data science solutions for its benefit.
The innovations that Data Science brings to sales mostly improve customer experience and as a result boost sales. Sales KPIs (Key Performance Indicators) and ROI (Return on Investment) may be enhanced with fewer efforts as well. Of course, to achieve this objective a great deal of data collection, processing, cleansing will be needed, but it is definitely worth the effort.
Comments (0)
Add a new comment: