Top 8 Data Science Use Cases in Marketing
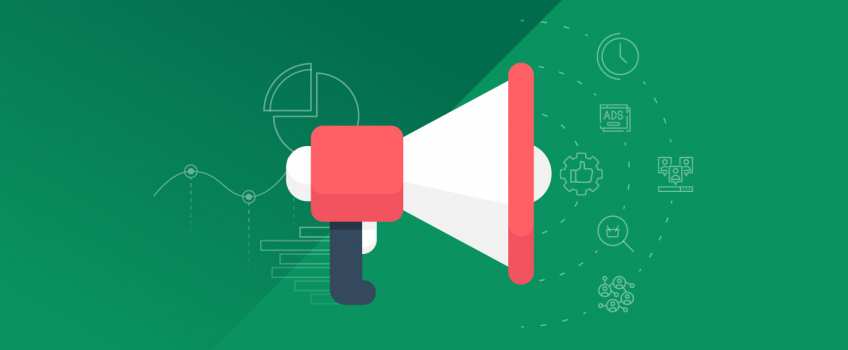
In this article, we want to highlight some key data science use cases in marketing.
As far as the key aim of data science is to turn data into actionable insights the marketing sphere cannot skip the application of these insights for its benefit. Big data in marketing provides an opportunity to understand the target audiences much better.
Data science is mostly applied in marketing areas of profiling, search engine optimization, customer engagement, responsiveness, real-time marketing campaigns. Moreover, new ways to apply data science and analytics in marketing emerge every day. Among these, the new use cases include digital advertising, micro-targeting, micro-segmentation, and many others.
Let us concentrate on several instances that present particular interest and managed to prove their efficiency in the course of time.
Customer segmentation
All customers are individuals. Therefore, a one-size-fits-all approach is not efficient at all. Customer segmentation comes to the rescue of the marketers in this case. Application of the statistical analysis allows marketers to slice the data and group customers.
Customer segmentation is a process of grouping customers into segments according to the coincidences of particular criteria in their characteristics.
There are three significant segmentation types that are the most often used. These are:
-
segmentation based on touchpoint engagement
-
segmentation based on purchase patterns.
Application of micro-segmentation appears to be a rising trend in marketing. Micro-segmentation is far more advanced. It helps to segment people into more precise categories especially concerning behavioral intentions. Thus, marketing actions may be tailored to the preferences even of the least numerous customer groups.
Real-time analytics
Real-time analytics proved to bring marketing insights into campaigns immediately. These real-time marketing opportunities become possible due to the recent boost in popularity of social media and communication technologies.
Efficient real-time analysis of data brings a considerable increase in revenues for the companies. Real-time algorithms work with two groups of data: customer data and operational data.
Customer data provides insights into customers’ wants, preferences, and needs. Operational data reflect various transactions, actions, and decisions made by the customers. Application of real-time data analysis brings efficiency, speed and high-performance rates to marketing campaigns.
Real-time analytics in marketing provides an opportunity to:
-
get more details about customers
-
find the efficient platforms
-
provide a unique customer experience
-
run real-time test
-
identify the best working practices
-
react and respond immediately.
Predictive analytics
At present, the data is easily accessible and available even for middle-size companies. This is why predictive analytics is so widely applied in marketing.
Predictive analytics is the application of statistical and machine learning algorithms to predict future with high probability. There are a lot of opportunities to apply predictive analytics in marketing. Let's consider those, which proved to be the most efficient.
Predictive analytics for customers' behavior
Cluster models, predictions, collaborative filtering, regression analysis are all applied to spot the correlation patterns in the customers' behavior to predict future tendencies in purchasing.
Predictive analytics to qualify and prioritize leads
Here belong predictive scoring, identification models and automated segmentation. These are related to qualifying and prioritizing leads to make your marketing efforts more effective. Applying these models, you can make sure that the most effective ready to purchase leads will get your call to action correctly.
Predictive analytics to bring the right product to the market
In this case, data visualization helps the marketing team to make the right decision about what product or service should be delivered to the market.
Predictive analytics for targeting
This is related to a whole bunch of predictive analytics models like affinity analysis, response modeling, churn analysis. These models are used to identify the highest value customers and address them with the right offer at the right time.
Recommendation engines
Recommendation engines are powerful tools in attempts to provide a personalized experience and high satisfaction rates to the customers. Marketers are those people who should pay particular attention to the application of the recommendation engines.
The key idea of the recommendation engines is to match the preferences of a customer with product features he or she might like. For this purpose, recommendation engines usually use the following models and algorithms: regression, decision tree, K-nearest neighbor, support vector machines, neural networks, etc.
Recommendation engines are a key targeted marketing tool for email and online marketing campaigns.
Market basket analysis
Market basket analysis refers to the unsupervised learning data mining techniques intended to learn the buying patterns and to disclose the co-occurrence relationships between purchases. Application of these techniques allows predicting future purchase decisions.
Moreover, market basket analysis can significantly improve the efficiency of the marketing message. Besides the type of the marketing message, whether it is direct offer, email, social media, phone call or newsletter you can offer the next best product suitable for a particular customer.
Optimization of marketing campaigns
The main task of the marketing team is to create an efficient, customer oriented, targeted marketing campaign dedicated to delivering the right message to the right people at the right time.
Optimization of marketing campaign involves the application of smart algorithms and models allowing to increase the efficiency. Modern technologies bring automation to the data collection and analysis process, reduce time spent on them, provide real-time results and spot the slightest changes in patterns. Smart data algorithms treat each customer individually. Thus, the high personalization level becomes more achievable.
The optimization process includes several steps that are equally important and require attention. Let us outline these steps:
-
Choose the right tools
Invest money in those tools that will efficiently gather and analyze data. Make sure the tools you choose can work together for the benefit of your campaign. Integrate the tools with existing systems and data.
-
Measure the metrics
Measuring metrics allows to identify processes and strategies that need improvement. Measure the parameters comparing them to your marketing goals.
-
Draw conclusions
Make right data-based decisions to make your marketing campaign as successful as possible.
Lead scoring
Customers' path through the sales funnel is staffed with various opportunities, options, and choices. Lead scoring is applied to identify those prospective customers who will go through the funnel and make their choice to the benefit of your product or service. What is the trick?
Lead scoring ranks the prospect according to a scale representing the value of each lead. The value of each lead may be identified differently, but often they are referred to as hot, warm or cold ones.
Lead scoring involves data collection concerning customers' demographics, responsiveness, purchase history, preferences, web page view, visits, likes, shares and even the type of e-mails they often react to.
As a result of lead scoring, the salespeople get qualified prospects regarding who is highly intended to buy. Thus, when products are offered to the right people, the sales boost.
Optimal campaign channels and content
The essence of all the marketing efforts is to reach the right customer. However, the marketing landscape has been changed and moved to the online world. Thus, the main task for the companies is to assure a strong online presence for the brand.
The leading part here is given to the selection of optimal digital marketing channels: email marketing, pay-per-click advertisement, search engine optimization, display advertising, Social Media Marketing, content marketing, affiliate marketing, online public relations. The choice is vast. To make this choice more comfortable, take the following steps:
-
define goals
-
allocate budget
-
determine your audience.
In its turn, a digital marketing challenge determines the type of content the brand can use. Blog posts, articles, videos, stories etc. All these types prove to be more or less effective depending on the channel used to distribute them.
Conclusion
The use cases mentioned above prove the statement that application of data science brings numerous benefits to marketing campaigns of various brands. Considering the amount of data available today it is essential not just to freeze it but to use it for the benefit of the company.
Transformation of data into meaningful insights is crucial for decision making. Our list of top data science use cases in marketing reveals specific features of data application in this area and real positive effects it may cause.
Comments (0)
Add a new comment: